Content Overview
Autonomous AI agents will change how groups do business by reading information, making choices, and carrying out tasks with minimum human input. These intelligent systems bring a new level of performance and adaptability to industries where they’d enable the automation of complex processes, enhanced productivity, and individualized experiences. Self-sufficient AI marketers are causing innovation in several industries-from optimizing supply chains to revolutionizing customer support. This article will look into what defines these marketers, their major differences from traditional automation, and the top providers supplying novel solutions.
Core Features of Autonomous AI Agents
Perception: Autonomous AI agents can collect information from different sources like sensors or digital inputs in order to understand their surroundings. For example, a chatbot agent gets an input from the user and perceives it as an intent.
Decision Making:

These agents employ Artificial Intelligence techniques, whether in the form of machine learning or even neural networks or reinforcement learning, for selecting the best actions.
Learning Ability:
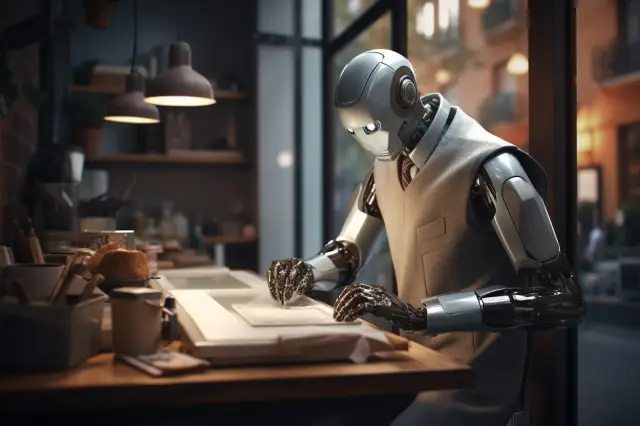
The agents are capable of learning and adjusting to new situations through algorithms to be better in the future.
Execution:
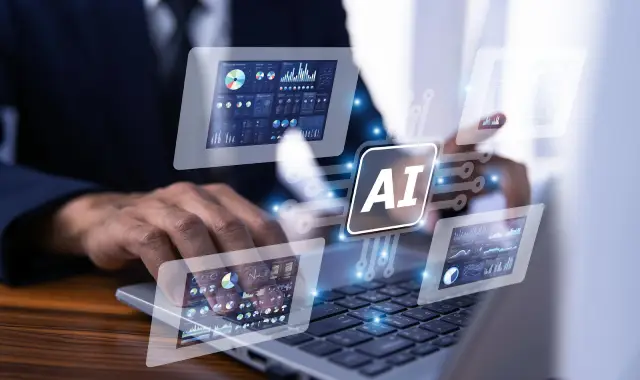
After taking a decision, agents will perform actions such as automating workflows, solving user queries, or even managing supply chains.
Real-World Applications:
Autonomous AI agents are changing industries:
- Healthcare: Helping in diagnosis and treatment recommendations.
- Finance: For investing and fraud detection.
- E-commerce: Intelligent chatbots to tailor customer experiences.
- Manufacturing: Improve production processes with predictive analytics.
What Is an Agent in Artificial Intelligence?
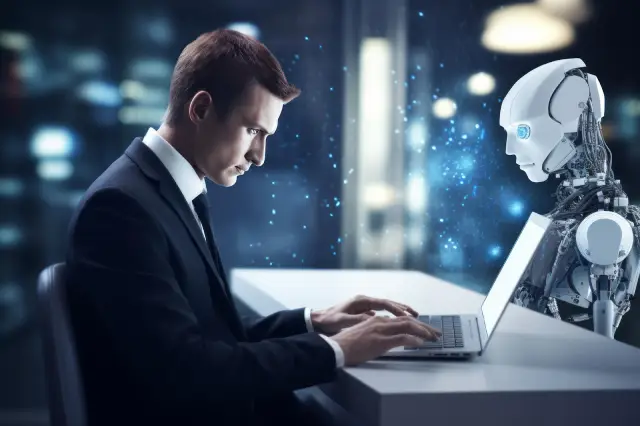
An agent in artificial intelligence is any system that can perceive its environment and act within it for achieving specific goals. The agents could differ in their complexity but are similar in that they always fulfill that one purpose: to act intelligently in any given context.
Main Components of an AI Agent:
- Sensors: They are the devices by which the agent perceives the environment. A good example is voice recognition for a virtual assistant.
- Actuators: These consist of what enables the agent to manipulate the environment, for instance, say, send messages or start workflows.
- Intelligence: It refers to the agent’s ability to process the inputs such that it can choose the best response or action possible.
AI agents vary in what they can do – some are very simple automated tools while others can take care of most tasks without human intervention and are sometimes even complicated.
Types of Agents in Artificial Intelligence
- Simple reflex agents:
- They run on rules predetermined before the execution.
- They react according to input, without any internal memory to think of a history or a relationship.
- Example-ac that switches on when the ambient temperature increases above a threshold.
- Model-based Agent:
As internal state or memory enables sensors to interpret what is happening in the environment as well as change the interpretation of an action depending on what happened before.
For example, navigation considering road conditions and events in past hours by a self-driving car. - Goal-based agents:
They carry out work based on the goals to be achieved so that the proofs of action for them are only those which contribute to the intended outcome.
Example-a search engine provides increasingly refined query results to produce most relevant answers. - Utility-Based Agents:
Make comparisons among various potential actions in order to maximize utility.
Example: E-commerce recommendation engines that suggest products based on user taste. - Learning agents:
Learn through the event in order to train their machine learning algorithms for improved performance.
Example: Virtual assistant technologies like Siri or Alexa, which learn the user’s behavior in time by recording the responses of those specific users.
Top Vendors Offering Autonomous AI Agents
1. OpenAI
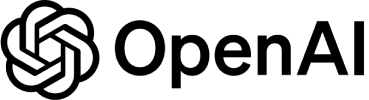
OpenAI is most known for the GPT models: an automated agent that enables marketing professionals to make decisions related to content generation and natural-language understanding. They are used with applications like virtual customer assistance, content generation, etc., which help organizations to automate some complicated processes with precision.
2. Google DeepMind
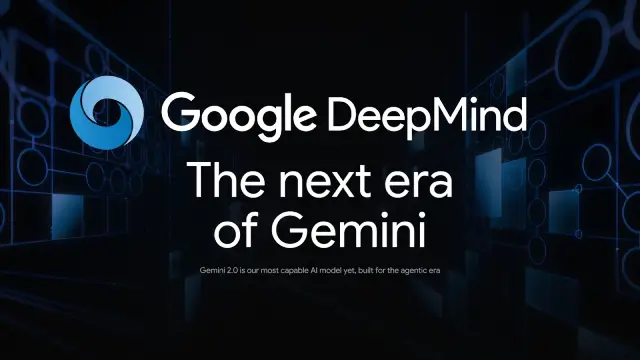
Google DeepMind started as an AI research company and then focused on developing abstractions of intelligent agents that solve complicated problems. By representing breakthroughs in protein folding research from AlphaFold to game-play cases in dynamic environment, solutions from DeepMind have shown extraordinary computational and adaptive capabilities.
3. IBM Watson
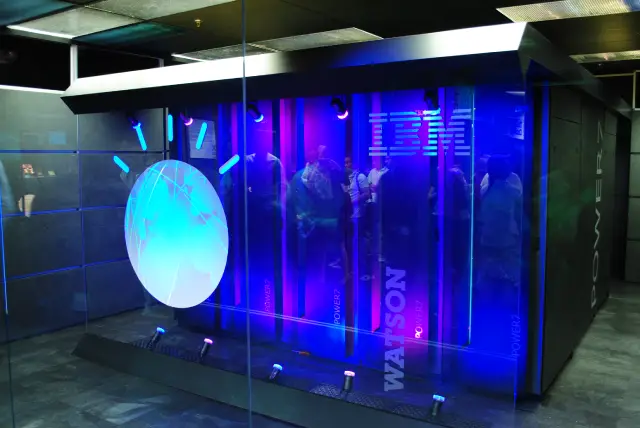
Offering self-sufficient agents for sectors such as health, finance, and retail, IBM Watson deals solely with enterprise-level AI-solutions. Watson agents spare action-oriented insight through natural language processing, deep learning, and decision-making capabilities to automate processes that involve advanced workflows.
4. Microsoft Azure AI

For developing and deploying intelligent agents customized to business objectives, Azure AI has pretty powerful tools, enabling conversational AI as well as process automation and advanced analytics, so it can empower businesses to integrate autonomous marketers seamlessly into their own operations.
5. Amazon Web Services (AWS)

AWS also has a complete range of AI and machine-learning tools, such as its SageMaker and Lex services, which support the creation of fully autonomous agents. These models allow businesses to completely automate customer interaction, process data, and control dynamic workflows.
Key Differences Between AI Agents & Traditional Chaining/RPA
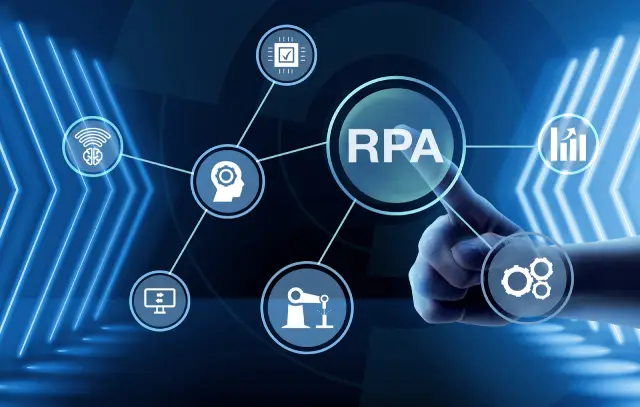
In actual fact, both AI dealers and old-fashioned forms of automation, such as chaining and Robotic Process Automation (RPA), are indeed intended for performing better and more efficient methods. However, the approaches and skills largely differ. For example, old chaining requires one process to follow predefined rules and workflow chains, but it would often need human handling of exceptional events. Similar to RPA, this technology concentrates on basal automation of redundant and repetitive tasks, for instance, data entries or generating invoices, keeping the element of no learning or adaptation to changes within the environment.
Accordingly, they connote the capabilities of autonomy, adaptability, and intelligence in comparison. Unlike the rule-based processes of RPA, AI dealers can be in a position to grasp their environment, analyze from experiences, and make decisions based on real-time information to deal with complicated unstructural tasks which can hardly be addressed through regular automation. For example, whereas RPA might automate the extraction of data from receipts, an AI agent might analyze shopping behaviors and suggest cost-saving strategies based on historical information. AI agents are transforming automation since they can adapt to rapidly changing environments and optimize procedures without continuous human intervention.
Applications of Autonomous AI Agents Across Industries
Healthcare
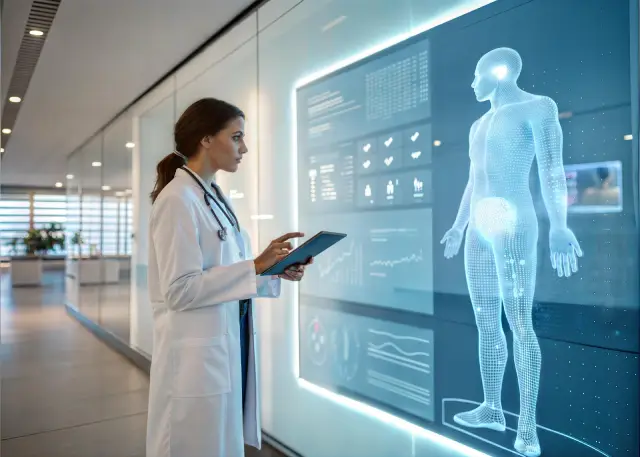
With technology, independent artificial intelligence marketers are using it in diagnosing ailments, treatments, and managing patient records. These tools analyze clinical photography to determine conditions such as high cancer accuracy. Virtual health assistants supervise patients at home, providing timely intervention and relieving medical specialists of their jobs.
Finance
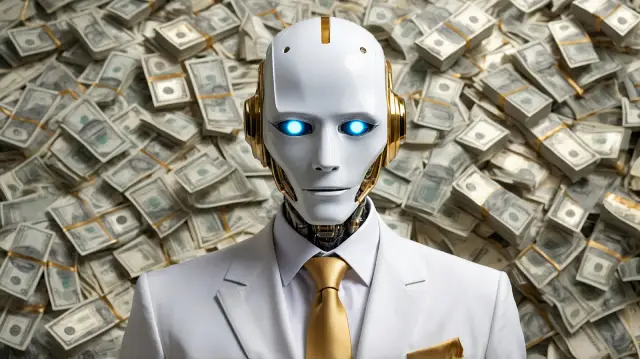
The economic sector will greatly benefit from self-sustaining AI agents in areas like fraud detection, portfolio management, and personal banking services. For instance, an AI agent can handle huge real-time transactional data and accurately detect fraudulent activities before they become infections. In treated investment management, agents optimized portfolios based on trends in the market, bringing higher returns on investment.
Supply Chain and Logistics

Independent AI agents predict demand, improve inventory, and automate logistics to gain efficiency in supply chain management. These agents rely on real-time data that makes instantaneous decisions such as rerouting shipments whenever needed to avert delays. Companies such as Amazon rely on these agents for managing warehouses and streamlining distribution systems.
E-Commerce
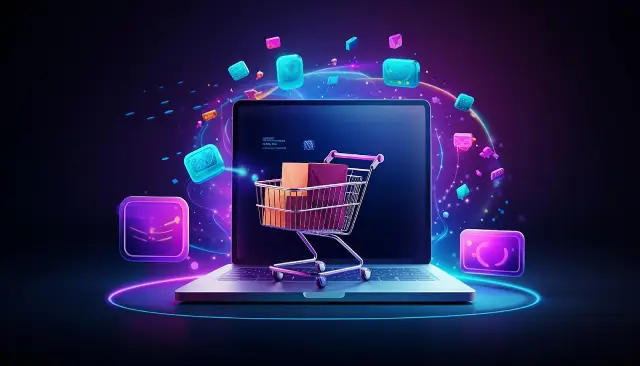
These E-trade platforms use self-governing artificial intelligence agents to cater personalized customer experiences. Agents recommend products based on surfing history, manage inventory, and optimize pricing strategies. AI-enhanced chatbots also further augment customer service by offering instant assistance and clarifying inquiries.
Manufacturing
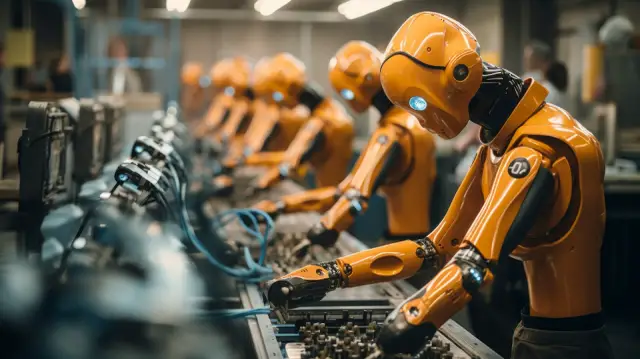
Manufacturing industries utilize autonomous AI agents to do predictive maintenance, quality control, and process optimization. AI agents predict possible failures by analyzing sensor data from machines, thus reducing downtime and costs. They also ensure consistent product quality by monitoring production processes in real time.
Ethical and Security Challenges of Autonomous AI Agents
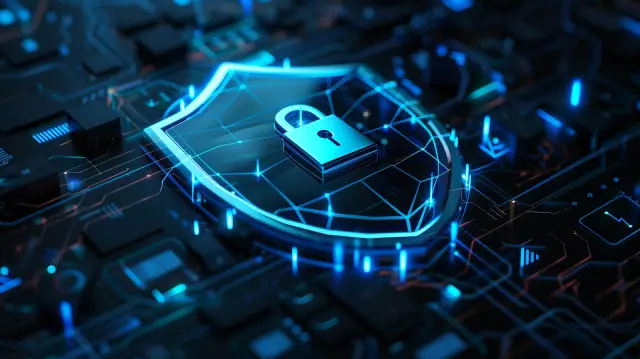
- Bias and Fairness
The AI retailers perform the research inadvertently and will mirror the bias present in the obtained data on which they are trained. This could lead to unbalanced consequences into some important programs such as hiring, lending, or even legal judgment. A preventive measure is to train retailers on diversified and neutral datasets. - Accountability
Difficulties arise if an agent becomes accountable for any fault of causing harm. Complexity lies within the question of whether the fault is imposed on the developers, the organization using the agent, or the agent itself. - Data Privacy
Most AI agents heavily rely on analyzing huge volumes of private and other sensitive data. Why then would someone want to secure this type of data for assurance in compliance with most of the data protection policies such as GDPR? - Security Risks
Artificial intelligence agents exist autonomously and are vulnerable to cyber-attacks and infiltration by malicious actors who can cause damage by coercing the agents into making harmful decisions. Perfect Conditions would spend well on cybersecurity measures aimed at protecting AI and the processes that these agents undergo within their operations.
Future Trends in Autonomous AI Agents
Higher Integration with IoT
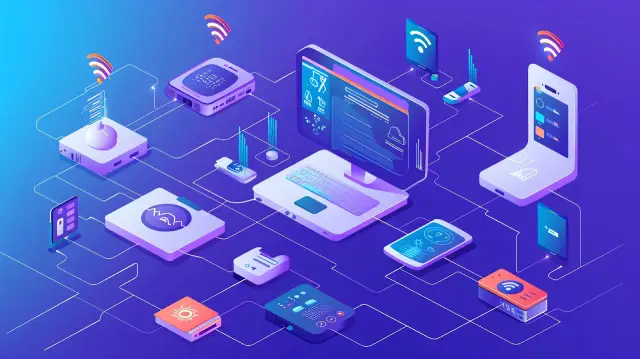
Smart ecosystems are turning out to be the Internet of Things (IoT) attached to independent AI agents. With respect to weather patterns, agents linked to IoT gadgets would make real-time decisions, including adjusting home thermostats or controlling traffic flow in smart cities.
NLP – Natural Language Processing Improvements

The continual advance of NLP would further make AI vendors sound more conversational and highly qualified in human grammar. Hence, interactions among customers and industries are becoming much more natural and intuitive.
Agents Self-Improving
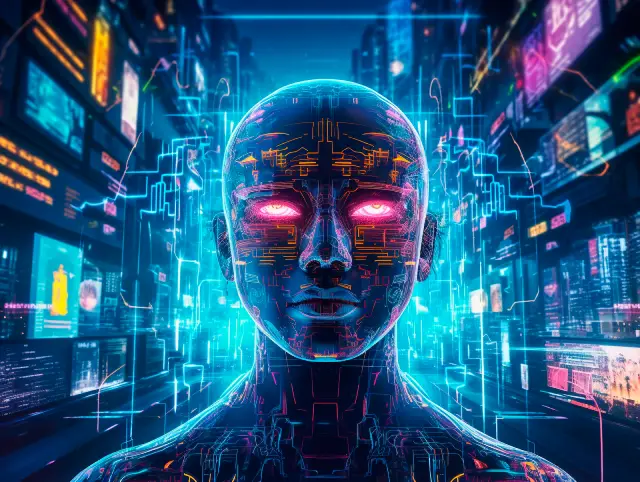
Reinforcement learning and other more sophisticated algorithms will probably allow agents that will be able to self-improve over time. These agents will not only be adaptive to their environments but will also devise entirely new methods to accomplish their goals.
New Market Penetration

Open and unexplored industries like education, legal services, and energy are now being accessed by self governing AI agents as the technology matures. From learning plans personalized for each student to legal research and energy management, its applications are limitless.
How Autonomous AI Agents Learn and Adapt
- Supervised Learning and Unsupervised Learning
Supervised education is when an Artificial Intelligence agent learns through classified data and the desired result is usually given. For instance, an autonomous agent for customer service might be taught through historical customer interactions and their resolutions. On the other hand, unsupervised learning is also helping agents to discover trends within the data without having previous labels. This is because it applies in market segmentation where the agent classifies customers according to their patterns in shopping behavior. - Reinforcement Learning
Reinforcement learning enables agents to act through negative or positive feedback based on the consequences of their actions. The given feedback can help them change their strategies. One example of reinforcement learning is allowing self-driving cars to learn most appropriate driving by simulating real-world situations. - Deep Learning and the Neural Networks
Deep learning increases the ability to process complex types of information, such as images, audio, and video. Neural networks or particularly deep neural networks help agents understand high-dimensional data and make valid predictions. Such capability is paramount in industries like health, where agents examine medical scans to identify diseases.
Wrap-Up
- Independent dealers function through the integration of belief, mastery, decision-making, and execution.
- There are many categories of dealers, which include simple reflex dealers and learning dealers, among others, for their specific purposes.
- The differences between AI sellers and regular chaining or RPA show the advanced capabilities of these sellers to deal with highly complex problems.
- These are some distinguished vendors such as OpenAI, IBM Watson, and Google DeepMind, all of which were at the forefront of bringing creative solutions into the field.
FAQs
What forms of retailers are synthetic intelligence?
AI marketers or agents are classified into simple reflex agents, model-based agents, goal-based agents, utility-based agents, and learning agents, each classified based on the kind of operation they perform and the complexity involved.
Who are the top companies providing autonomous AI marketers?
The leading suppliers include OpenAI (for GPT-based models), Google DeepMind (for research-centric agents), IBM Watson (for enterprise solutions), Microsoft Azure AI (for enterprise automation tools), and Amazon Web Services (AWS) that serve AI frameworks like SageMaker and Lex.
What are the complexities about operating independent AI marketers?
The core dilemmas involve securing data privacy and independence in decision making, as well accountability and guarding against risks due to security failure.
What are the future trends in autonomous AI agents?
These include greater integration with IoT, better natural language processing technology, self-improving agents, and the addition of education, law, and energy to their growing number of sectors.
What are autonomous AI agents?
The surreal self-reliant AI retailers What the autonomous AI traders, who self-design analyze, decide, and execute, require is a least amount of human input. Such systems bring efficiency, flexibility, and automation to industries.
What distinguishes a self-sufficient AI agent from traditional automation methods?
Like static process flows, traditional automation lacks self-governing AI agents that can study, adapt, and make decisions in dynamic environments without the constant need for human oversight.
In which industries will you find self-sufficient AI marketers?
Self-sustaining AI sellers prove a huge boon for industries such as health care, finance, e-trade, manufacturing, and logistics by improving efficiency, automating processes, and offering personalized services.
What are the core features of self-sufficient AI agents?
Some central ingredients of self-sufficient AI agents include perception (data collection), decision-making (using AI techniques), learning capability (adapt via algorithms), and execution (automating tasks).